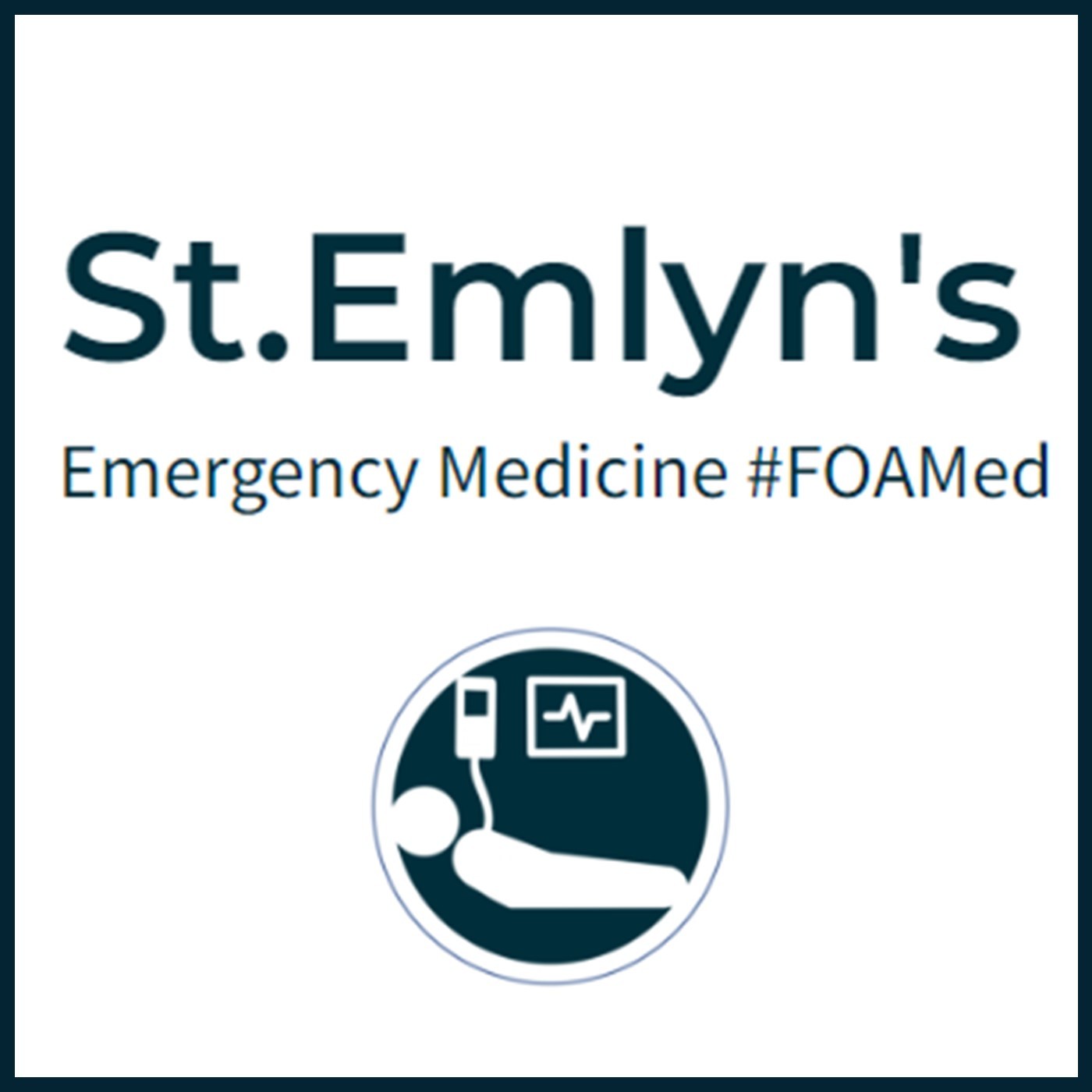
1.3M
Downloads
272
Episodes
A UK based Emergency Medicine podcast for anyone who works in emergency care. The St Emlyn ’s team are all passionate educators and clinicians who strive to bring you the best evidence based education. Our four pillars of learning are evidence-based medicine, clinical excellence, personal development and the philosophical overview of emergency care. We have a strong academic faculty and reputation for high quality education presented through multimedia platforms and articles. St Emlyn’s is a name given to a fictionalised emergency care system. This online clinical space is designed to allow clinical care to be discussed without compromising the safety or confidentiality of patients or clinicians.
Episodes
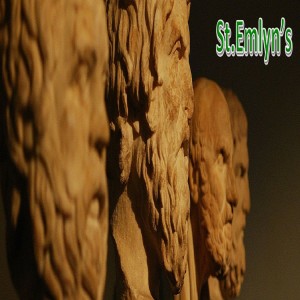
Thursday Sep 15, 2016
Ep 79 - Critical Appraisal Nugget: Selection Bias
Thursday Sep 15, 2016
Thursday Sep 15, 2016
Summary of Selection Bias in Medical Research
Introduction
Selection bias is a critical issue in medical research that can undermine the validity of study findings. It occurs when there is a systematic difference between the study population and the broader population the research aims to represent. Understanding selection bias is essential for clinicians and researchers, as it can lead to questionable conclusions and affect clinical practice. This summary covers the definition of selection bias, its sources, and ways to mitigate it, along with a case study illustrating its impact.
What is Selection Bias?
Selection bias happens when the participants in a study do not accurately reflect the general population. This discrepancy can result from various factors, including how patients are selected, the setting of the study, and the timing of patient recruitment. Such biases can skew research results, making them less applicable to real-world situations. As medical professionals rely heavily on research to inform clinical decisions, recognizing and addressing selection bias is crucial.
Sources of Selection Bias
-
Study Environment
The environment where a study is conducted can significantly influence patient selection. For instance, patients in a general practitioner's office might have a lower prevalence of serious conditions compared to those in an emergency department. Additionally, studies in specialized tertiary care centers often include patients with more severe or rare conditions, which may not represent the general patient population. This can lead to overestimating or underestimating the effectiveness of treatments or the accuracy of diagnostic tests.
-
Timing of Patient Recruitment
The timing of patient recruitment is another source of selection bias. The stage of illness at which patients are recruited can affect study outcomes, especially in diagnostic studies. For example, the diagnostic value of CRP for appendicitis changes depending on when it is measured. Additionally, certain conditions may present differently depending on the time of day or week, potentially leading to an incomplete understanding of a condition's prevalence or severity if the study only includes patients from specific times.
-
Retrospective vs. Prospective Studies
Retrospective studies, which rely on historical data, are particularly vulnerable to selection bias. These studies may selectively include data from periods with better patient outcomes, leading to skewed results. They may also suffer from incomplete data or changes in diagnostic criteria over time, making it difficult to generalize findings. Prospective studies, while more controlled, also need careful planning to avoid selection bias, especially in defining inclusion and exclusion criteria.
-
Convenience Sampling
Convenience sampling involves selecting patients based on availability rather than a structured protocol, often due to resource limitations. This can result in a non-representative sample, such as including only daytime patients who might differ from those presenting at night. While convenience sampling can be a pragmatic choice, it often leads to underrepresentation of certain patient groups, potentially biasing study findings.
Mitigating Selection Bias
To mitigate selection bias, researchers should strive for comprehensive sampling strategies, such as random or consecutive sampling. Where complete sampling is not possible, they should transparently report potential biases and the measures taken to minimize them. For instance, using screening logs or adjusting for demographic differences can help address disparities between recruited and non-recruited patients. Sensitivity analyses can also be used to understand the impact of excluding certain patient groups.
Case Study: Thrombolysis in PEA Cardiac Arrest
A recent journal club discussion highlighted a retrospective cohort study by Shereefi et al., examining the efficacy of half-dose thrombolysis in patients with PEA cardiac arrest and confirmed pulmonary embolism (PE). The study raised concerns due to several potential biases. The arbitrary selection of a 23-month inclusion period, without a clear rationale, suggests the possibility of survival bias, as it included only patients who survived long enough to receive a confirmatory diagnosis of PE. This selective inclusion means the findings might overestimate the treatment's effectiveness, as the study only considered patients with a relatively favorable prognosis.
Moreover, the study's setting in a specialized environment and the inclusion of only confirmed PE cases limit the generalizability of the results. In practice, thrombolysis may be administered based on clinical suspicion rather than confirmed diagnosis, which involves a broader and potentially more diverse patient group. The study's focus on survivors also excludes those who may have died before a diagnosis, further skewing the data towards more favorable outcomes.
Implications of Selection Bias
Selection bias can significantly impact the interpretation of study results and, consequently, clinical decisions. It can lead to over- or underestimation of a treatment's effectiveness or the prevalence of a condition. This bias can also affect healthcare policy and practice guidelines, potentially disadvantaging underrepresented patient groups. For example, guidelines developed from biased research may fail to address the needs of older adults or those with comorbidities if these groups are underrepresented in studies.
Conclusion
Selection bias is a pervasive issue that can undermine the credibility of medical research. It arises from various sources, including the study environment, timing of recruitment, study design, and sampling methods. While complete elimination of selection bias is challenging, awareness and careful methodological design can mitigate its effects. Researchers and clinicians must critically appraise studies, considering potential biases and their implications for clinical practice. By doing so, we can make more informed decisions and improve patient care. At St. Emlyns, we continue to explore these critical appraisal topics to support evidence-based practice.
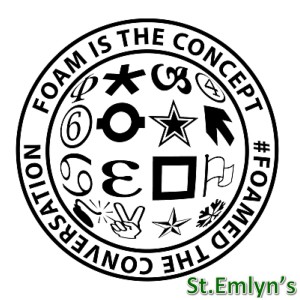
Wednesday Aug 24, 2016
Ep 77 - Critical Appraisal Nugget 2: Blinding and Masking in clinical trials
Wednesday Aug 24, 2016
Wednesday Aug 24, 2016
Understanding Blinding in Clinical Trials: A Comprehensive Guide
Blinding, also referred to as masking, is a crucial methodological approach used in clinical trials to eliminate bias and ensure the validity of study outcomes. This practice involves keeping study participants, clinicians, and researchers unaware of the treatment assignments. The goal is to prevent the knowledge of treatment allocation from influencing behaviors, perceptions, or evaluations, thus maintaining the integrity of the research.
Types of Blinding in Clinical Trials
Single Blinding: In this approach, only the participants are unaware of the treatment they receive. For example, in a drug trial comparing an anticoagulant to a placebo, participants do not know which they are receiving. This method helps prevent the placebo effect or other biases arising from participants' expectations. Ensuring effective single blinding requires that the placebo and active treatment appear identical in all sensory aspects, including appearance, taste, and even side effects.
Double Blinding: This method extends the concealment to both participants and the clinicians or researchers administering the treatment. It is crucial because knowledge of the treatment can influence the clinical management of the patient or the interpretation of symptoms. For example, if a doctor knows a patient is receiving a placebo, they might provide less attentive care. Double blinding helps ensure that all patients receive consistent care, and it prevents clinicians from unintentionally influencing the outcomes based on their expectations.
Triple Blinding: The most comprehensive form of blinding, triple blinding, includes blinding the data analysts as well. This method prevents bias during the interpretation of study results, as analysts are unaware of which group received the treatment. This approach is particularly important when the data analysis involves subjective judgments or when the analysis plan is not strictly predefined. Triple blinding helps ensure that data is handled objectively, leading to more reliable conclusions.
Importance of Blinding in Clinical Trials
Blinding is essential to minimize various forms of bias that can compromise the validity of clinical trials. These biases include:
-
Participant Bias: When participants know the treatment they are receiving, it can influence their reporting of symptoms and outcomes, skewing the study results.
-
Observer Bias: Clinicians and researchers may consciously or unconsciously alter their assessments based on their knowledge of the treatment allocation. This can affect how symptoms are recorded or how interventions are implemented, potentially leading to biased outcomes.
-
Analyst Bias: Data analysts may be influenced by their expectations or hypotheses if they know which treatment group participants belong to. This can affect the objectivity of the data analysis, making the results less reliable.
Practical Challenges in Blinding
Despite its importance, blinding is not always feasible or ethical in all types of studies. For example, in trials comparing physical interventions like a wrist splint versus a plaster cast, it is impossible to blind participants or clinicians due to the visible nature of the treatments. In such cases, researchers must adopt a pragmatic approach, using rigorous randomization and transparent reporting to mitigate potential biases.
A notable case highlighting the challenges of blinding is a study conducted by Dan Horner and colleagues on the treatment of calf deep vein thrombosis (DVT). The study compared the use of warfarin with no anticoagulation. Ideally, the study would have included a placebo group, but ethical and practical concerns made this impossible. Patients on warfarin require regular monitoring and dose adjustments, which could not ethically be simulated for a placebo group. As a result, the study was conducted without blinding but still provided valuable insights into the treatment's potential benefits.
Sham Interventions in Blinding
In some studies, particularly those involving surgical procedures, sham interventions are used to maintain blinding. This involves performing procedures on the control group that mimic the intervention without providing therapeutic benefits. For instance, in trials assessing surgical techniques, control group participants might undergo anesthesia and incision without actual surgery. This method is ethically sensitive but can be crucial in preventing bias from influencing the study's outcomes.
Blinding in Diagnostic Studies
Blinding is also vital in diagnostic studies, where it ensures that the assessment of diagnostic tests is not influenced by pre-existing knowledge about the patients. For instance, in evaluating a new diagnostic test like a D-dimer test for pulmonary embolism, blinding the interpreters of test results is essential. It prevents bias in the interpretation of test outcomes, ensuring that results are evaluated based on the test's performance rather than expectations or assumptions.
Conclusion
Blinding is a fundamental component of clinical trial design, crucial for minimizing bias and ensuring the validity of study findings. Whether it is single, double, or triple blinding, the technique helps maintain objectivity in clinical research, making the results more reliable and trustworthy. While blinding is not always possible, especially in trials involving visible interventions, researchers must strive to minimize bias through careful study design and transparent reporting.
As readers and critical appraisers of clinical research, it is essential to look for evidence of blinding and consider its impact on the validity of study results. Understanding the importance and challenges of blinding can help us better interpret clinical trial outcomes and make informed decisions in healthcare.
Thank you for joining us at St. Emlyn's. Stay curious, critically appraise the evidence, and continue exploring the fascinating world of clinical trials. Until next time, stay informed and engaged in advancing medical knowledge.
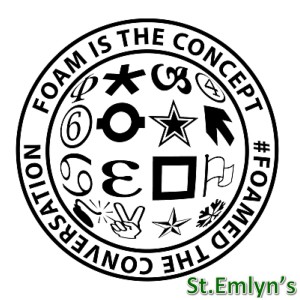
Wednesday Aug 10, 2016
Ep 75 - Critical Appraisal Nugget 1: Randomisation
Wednesday Aug 10, 2016
Wednesday Aug 10, 2016
Understanding Randomization in Clinical Trials: A Guide for Critical Appraisal
Welcome to the St. Emlyn's blog, your go-to resource for insights into emergency medicine and critical care. Today, we're diving into a crucial aspect of clinical research: randomization. Whether you're preparing for exams like the FR-KEM or just want to deepen your understanding of clinical trials, understanding randomization is key to critical appraisal. This blog post will walk you through the essentials, common pitfalls, and best practices for ensuring robust study design.
What is Randomization?
Randomization is a foundational process in clinical trials, particularly those evaluating interventions. It refers to the random allocation of participants into different treatment groups. This process aims to eliminate selection bias and ensure that differences in outcomes can be attributed to the intervention itself rather than other factors.
Why is Randomization Important?
Randomization is crucial because it helps establish causality. Without it, studies might only reveal associations rather than true cause-and-effect relationships. For example, if we observe patients receiving different treatments in a non-randomized manner, systematic differences between the groups—such as varying standards of care—could confound the results. Randomization seeks to balance these factors, allowing for a clearer interpretation of the intervention's effectiveness.
Key Components of Randomization
-
Random Allocation: This is the process of assigning participants to treatment groups purely by chance. It can be done using random number tables, computer-generated sequences, or other methods that ensure allocation is not influenced by investigators or participants.
-
Allocation Concealment: This involves hiding the allocation sequence from those involved in enrolling participants. It's vital to prevent selection bias, where researchers might consciously or unconsciously influence the assignment of participants to specific groups.
-
Blinding: While not a part of randomization per se, blinding is closely related. It refers to keeping participants, healthcare providers, and researchers unaware of which treatment group participants are in. This prevents performance and detection biases.
Common Pitfalls in Randomization
Despite its importance, randomization can be implemented poorly, leading to biased results. Here are some common pitfalls:
-
Inadequate Randomization Methods: Methods like assigning treatments based on birth dates or day of the week might seem random but can introduce systematic biases. For instance, there could be differences in care based on the day or time, making these methods unreliable.
-
Failure to Conceal Allocation: In the past, brown envelope methods were used, where the treatment assignment was sealed in an envelope. However, this method is vulnerable to tampering. For instance, researchers might be tempted to "peek" at the assignment and selectively enroll participants, compromising the study's integrity.
-
Small Sample Sizes: Small trials are particularly vulnerable to imbalance in baseline characteristics between groups purely by chance. This can lead to skewed results that do not accurately reflect the intervention's efficacy.
Best Practices in Randomization
To ensure robust and reliable results, certain best practices should be followed:
-
Use of Reliable Randomization Methods: In modern trials, computer-generated random numbers are the gold standard. They provide true randomness and can be tailored to the specific needs of the study.
-
Allocation Concealment Techniques: More sophisticated methods like centralized randomization, where a third party manages the allocation process, can help maintain concealment. In some studies, web-based or voice-based systems are used, which provide real-time allocation while preventing researchers from manipulating the process.
-
Stratification and Block Randomization: To address the issue of unequal distribution of participants' characteristics, stratification and block randomization are employed. Stratification involves grouping participants based on certain characteristics (e.g., disease severity) and ensuring even distribution across treatment groups. Block randomization, on the other hand, ensures that each treatment group has an equal number of participants within defined blocks, maintaining balance throughout the study.
The Role of Randomization in Analyzing Results
When analyzing the results of a randomized controlled trial (RCT), the first step is to examine the baseline characteristics of the treatment groups. This is often presented in Table 1 of a study. The purpose is to ensure that randomization has successfully created comparable groups. If significant differences exist, they could confound the results, making it harder to attribute outcomes to the intervention alone.
Another critical aspect is to consider the size of the trial. Larger studies are generally better at balancing characteristics between groups, reducing the likelihood of chance imbalances. However, even in well-randomized studies, it's possible for imbalances to occur, especially in smaller trials. Researchers must acknowledge these potential imbalances and adjust their analyses accordingly.
Practical Considerations in Emergency Medicine
In emergency medicine, the need for rapid, reliable randomization methods is particularly pressing. Web-based randomization systems offer a convenient solution, providing quick, secure, and tamper-proof allocation. Similarly, voice-based systems, where a computer assigns treatment groups via a phone call, are another practical option.
For those conducting smaller trials, there are accessible tools available, such as Sealed Envelope (sealedenvelope.com), which offers randomization services tailored to smaller studies. These tools help maintain the integrity of the randomization process, even in resource-limited settings.
Special Considerations: Trials with Diverse Populations
In clinical trials, particularly in emergency settings, researchers often encounter a wide range of patient severities. For instance, in head injury studies, patients can vary significantly in their Glasgow Coma Scale (GCS) scores. In such cases, simple randomization may inadvertently group all severe cases into one treatment arm, skewing the results.
To mitigate this, researchers use stratification, ensuring that key subgroups (e.g., GCS < 8) are evenly represented across treatment groups. This not only improves the internal validity of the study but also enhances the power of the statistical analyses, providing more reliable results.
Advanced Randomization Techniques
As trials become more complex, so do the randomization techniques. Block randomization is one such method that ensures each treatment group receives participants throughout the study, rather than in uneven waves. For example, rather than having all participants receive treatment A first, followed by treatment B, block randomization allocates treatments in smaller blocks (e.g., groups of 20), maintaining balance throughout.
This method is particularly valuable in trials with interim analyses or those that may stop early due to significant findings. It ensures that at any given point, the distribution of participants is roughly equal, allowing for fair and accurate assessment of the treatment effects.
Conclusion: The Importance of Rigorous Randomization
Randomization is the cornerstone of robust clinical trial design. It minimizes biases, balances baseline characteristics, and supports the validity of causal inferences. However, the process must be meticulously planned and executed. From choosing the right method to ensuring allocation concealment, every step is crucial in maintaining the integrity of the study.
For clinicians and researchers, understanding the nuances of randomization helps in critically appraising literature and designing their own studies. Whether you're preparing for an exam or conducting a trial, appreciating the intricacies of randomization will enhance your ability to interpret and apply clinical research findings effectively.
At St. Emlyn's, we emphasize the importance of thorough critical appraisal skills. By mastering these concepts, you'll be better equipped to discern high-quality evidence and make informed decisions in your clinical practice. Stay tuned for more insights and practical tips on navigating the world of clinical research.